How to Ensure Data Quality for an Analytics Application?
Data quality is of paramount importance for an analytics application. Poor quality data can not only be misleading but also potentially dangerous. Data-driven decisions can get hampered and business intelligence (BI) gets affected if data quality is not maintained.
But the truth is that data quality issue was among the top three problems of BI and analytics users every year in The BI Survey since it was first started in 2002. If the situation is so dismal and its ramifications so profound, it makes sense to look at ways and means of remedy.
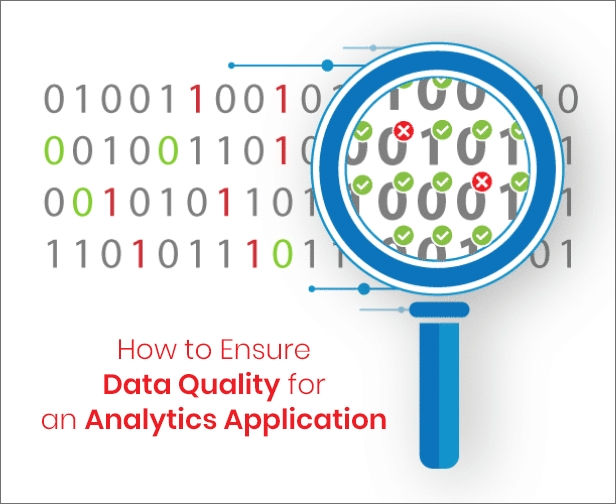
This post talks about actionable ways by which you can maintain high quality in the data pipelines of your analytics applications.
How Can we Define “Data Quality”?
Data can be said to be of good quality when it fulfils its dependent processes and becomes useful for its intended clients, end-users, and applications. Data quality impacts business decisions, regulatory mechanisms, and operational capacities.
There are five parameters by which we can determine data quality:
Accuracy: Is your data accurate and collected from reliable sources?
Relevancy: Is the data able to fulfil its intended use?
Completeness: Are all data records and values complete?
Timeliness: Is the data fresh up to the last minute, especially for time-sensitive processes?
Consistency: Can you cross-reference data from multiple sources? Is its format compatible with the dependent processes or applications?
How Can We Maintain Data Quality?
For an analytics application, data quality is non-negotiable. There should be a zero-tolerance policy for errors in data pipelines because this can impact the credibility and performance of analytics applications.
In such programs, data is the raw material based on which deductions are made and decisions are taken. If the raw material itself is sub-standard, the outcome will also be of poor quality. Hence, data quality in analytics applications should be a priority.
Below, we have outlined best practices and actionable steps by which you can improve and maintain data quality in your applications:
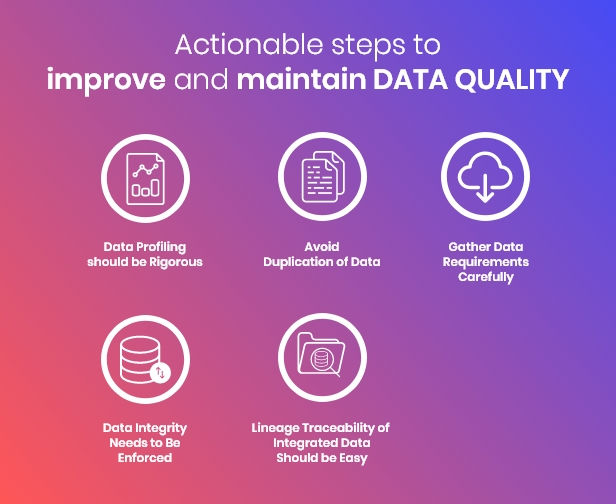
1. Data Profiling should be Rigorous
Often, data is collected by third parties or is submitted by multiple sources, which can be a reason for its dubious nature. In such cases, quality of data is not guaranteed and a data profiling tool becomes essential.
A data profiling tool should check the following data aspects:
Format and patterns of data
Consistency between data records
Anomalies in data distribution
Data completeness
Data profiling should be automated and constant. You should configure alerts for instances when errors are detected. A dashboard with KPI metrics of data profiling should be maintained.
2. Avoid Duplication of Data
“Duplicate data” means the same content as an existing data set, in full or partial. Such data sets are generated by different people for different purposes and applications, but their content is exactly the same. The data set is collected from the same source and by using the same collection logic.
The problem with duplicate data is that it creates a cascading effect on all the applications that are using it. If a data source gets corrupted or a collection logic is erroneous, all the duplicate sets get affected and this can impair all the processes that involve the data set. It becomes difficult to remedy all the affected processes and track the source of leakage.
To avert this risk, you need to establish fool-proof data pipelines from start to finish. Data modeling and architecture of each pipeline needs to be cautiously designed. Have a data governance system in place. Manage data in a unified, centralized system.
You also need robust communication systems so that cross-functional teams can get a ring side view of what the other teams are doing. If they detect any data duplication issues, they can raise an alarm instantly and issues can be capped at the origin itself.
3. Gather Data Requirements Carefully
You should document all data use cases, preferably with examples and visualization scenarios. Try to present data accurately. Communicate clearly with the client about their expectations from data discovery.
Keep all data requirements neatly filed and in a shareable mode so that the entire team can access them. You need business analysts in your development team because they understand perspectives of both sides, namely client and developers. They also perform analysis of the impact that data requirements will have, plus create A/B tests to check all app iterations.
4. Data Integrity Needs to Be Enforced
You need data integrity measures such as triggers and foreign keys for your analytics application. As data sources multiply, you need to house them in multiple locations. Then, you have to reference data sets relatively. Data integrity ensures this referential system is error-proof.
They corroborate processes with best practices of data governance. With the advent of Big data, referential enforcement has become a complex yet essential module. Without it, your data can be outdated, delayed, or erratic.
5. Lineage Traceability of Integrated Data Should be Easy
When data sets feed into each other, error in one record can offset a chain of errors that can paralyze the entire application. If you build lineage traceability, you can trace the origin of an error quickly and save impending disaster.
There are two aspects of this process: meta-data and data itself. In the former case, tracing is done by following relationships existing between records and fields. In the latter, you can drill down upon the exact data that has been compromised.
Meta-data traceability should be factored in during the data pipeline design stage itself. It is much easier to sift through meta-data than tons of data records. Hence, this mode should be incorporated in your app’s data governance policy.
Conclusion
You need to empower your data control teams to interweave best practices of data quality within your app’s architecture. It is important that you understand that your analytics app will be of no use to end-clients if its data quality is not pixel-perfect.
Hope you found the post informative. Let us know about the data quality best practices you follow.
To know more about iView Labs, kindly log on to our website www.iviewlabs.com and to get in touch with us with your queries and needs just write us an email on info@iviewlabs.com and sales@iviewlabs.com. Download the latest portfolio to see our work.
Comments